
In terms of our ability to use numbers to drive decisions, we’ve come a long way from using Excel spreadsheets. When Google Analytics was first introduced, it was a way for everyone to understand their website’s performance without needing to gather data manually. In just over fifteen years, we have tools today that don’t just gather data and collate it, but also use it to share highly actionable, business-specific insights and recommendations.
In the domain of data analytics, there are four types of analytics models- descriptive, diagnostic, predictive and prescriptive. Let us understand how each of these analytical models are relevant for an eCommerce brand, and how to understand the insights that they offer.
Each of these analytics models has a role to play. Descriptive Analytics can tell you what happened in the past, and is therefore the first step in any analytics-based intervention. Diagnostic Analytics, on the other hand, helps you understand why something happened in the past.
Forecasting is vital to the growth of any retail business. Predictive Analytics predicts what is most likely to happen in the future based on past trends and market forces. Prescriptive Analytics recommends actions you can take to affect those outcomes, and precisely which action can cause what outcome.
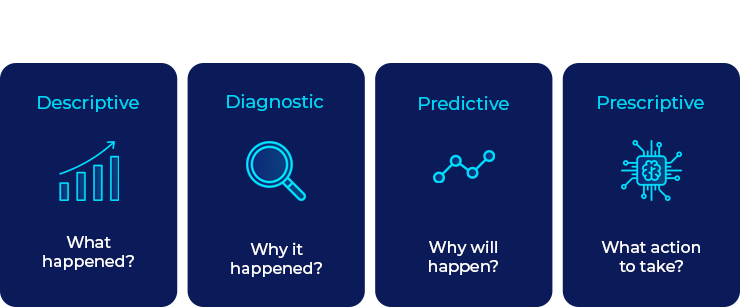
1. Descriptive Analytics
Descriptive analytics can help a brand understand if there is a problem within the system, and what that problem is. It typically uses Key Performance Indicators (KPIs) such as revenues, ROI, conversion rates etc to detect the performance of the system compared to a previous time period. It looks at historical data to detect anomalies and uses visualization tools to represent the same. On Graas you can see this in the form of different types of dashboards.
2. Diagnostic Analytics
This is a domain of advanced analytics, where machine leaning algorithms and AI are used to assess and deliver an explanation for the cause of a particular problem in a system. Through data recovery, correlations and drill down, diagnostic analytics answer the question - “Why did it happen?”
Diagnostic analytics uses key data from several sources and establishes a cause-and-effect relationship between multiple events to help brands understand the prime cause for a problem.
On Graas, you can see this in the form of insights. Diagnostic analytics can help brands identify causes for a variety of effects and offers multiple explanations, for instance:
Why did yesterday’s revenue decrease compared to the previous day?
Possible reason 1 for the above-mentioned decrease - Yesterday's Conversion rate for Device - DESKTOP Decreased by X% compared to the day before yesterday.
Possible reason 2 for the above-mentioned decrease - Yesterday's Revenue for Campaign - ABC Decreased by Y% compared to the day before yesterday.
Graas identifies these focused insights and segregates them into different categories based on the geography of the customers, type of user, type of campaign, type of devices, and more.
3. Predictive Analytics
Predictive analytics uses data gathered and the conclusions it offers to further predict what actions in the future would have what consequences.
In a retail context, it presents insights such as, “based on the past week’s data, you product XYZ is likely to go out of stock in the next 3 days.”
4. Prescriptive Analytics
In this newest frontier in analytics, algorithms not only understand the problem at hand, but also deliver a solution to be implemented in the future, from a series of potentially good solutions. The AI engine uses simulations, event processing, and graph analysis to determine the best course of action.
It answers the question, “what is the right decision to make for my particular context and at this particular time?”
On Graas, these are visible as recommendations. For example, the AI engine may recommend that you decrease the budget of a campaign by 50% if it isn’t performing as well as it should. It can also provide you with user experience insights and inventory level insights, such as asking that you add stock for a certain SKU, or that Coupon Codes need to be displayed at Checkout for easier usage.
Such real-time data-backed and mapped insights recommendations help brands make informed decisions and target specific issues with specific solutions.
Why should you choose Graas?
Graas is a data-driven recommendation engine that helps brands understand and consolidate their demand generation and demand fulfilment efforts based on a myriad of factors. It leverages data inputs from multiple sources to assess the performance and goals of the brand and delivers actionable insights to help comprehend, strategize and find solutions for a problem.
Explore Graas to leverage data like never before, and enhance sales for your eCommerce brand. Please fill in your details and experience a platform free trial here.
Commentaires